
Machine Learning Morphisms
Machine Learning Morphisms (MLM) are a mathematical framework to describe Machine Learning Applications. They are designed to encode as many operations as possible, from preprocessing to feature engineering to model training.
​
MLM's can mathematically be described as a 5-tuple:
​
-
Input probability space
-
Output probability space
-
Learning morphism
-
Prior distribution on parameters
-
Empirical risk function
​
The parameters are learned by optimizing the empirical risk function over a set of data.
​
The key idea with MLM's is the idea of composition which allows us to chain together operations and represent a workflow as a single mathematical object. This allows for potential joint optimization and modular design.
​
As an application, I built a machine learning model to predict 30-Day hospital readmissions. This model incorporates the TDA Mapper algorithm in a novel way to create an ensemble model that improves over the current tools used by Barnes Jewish Hospital.
​
A full description of MLM's can be found here.
​
A set of properties and operations used to design MLM's:
-
Asymptotic Equality: Concept of equality of two workflows based on the expected risk.
-
Structural Composition: Joint Optimization of Parameters.
-
Output Composition: Sequential Optimization of Parameters.
-
Workflow: Sequence of compositions.
-
Separability: Parameters can be learned by solving smaller dimensional Problems.
​
Publications:
[1]: E. Cawi, P. S. La Rosa, and A. Nehorai, "Designing machine learning workflows with an application to topological data analysis," PLOS ONE, Vol. 14, No. 12, pp. 1-26, Dec. 2019.
[2]: A. C. Tukpah, E. Cawi (Co-First), L. Wolf, A. Nehorai; L. Cummings-Vaughn (2020), "Development of an Institution Specific Readmission Risk PredictionModel for Real-Time Prediction and Patient-Centered Interventions",in revision for Journal of General Internal Medicine, 2020.
[3]: E. Cawi, P.S. La Rosa, and A. Nehorai, "Conditions for Separability of Machine Learning Workflows", submitted, Journal of Artficial Intelligence Research
[4]: E. Cawi, P.S. La rosa, and A. Nehorai, "Covariance Bounds for Machine Learning Workflows" (Working title), in preparation.
​
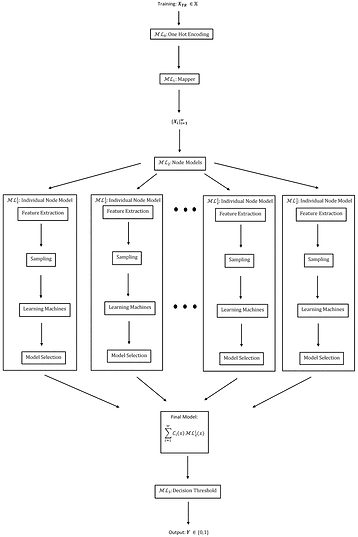